Segmenting is the process of putting customers into groups based on similarities,
and clustering is the process of finding similarities in customers so that they can be grouped, and therefore segmented. They seem quite similar, but they are not quite the same.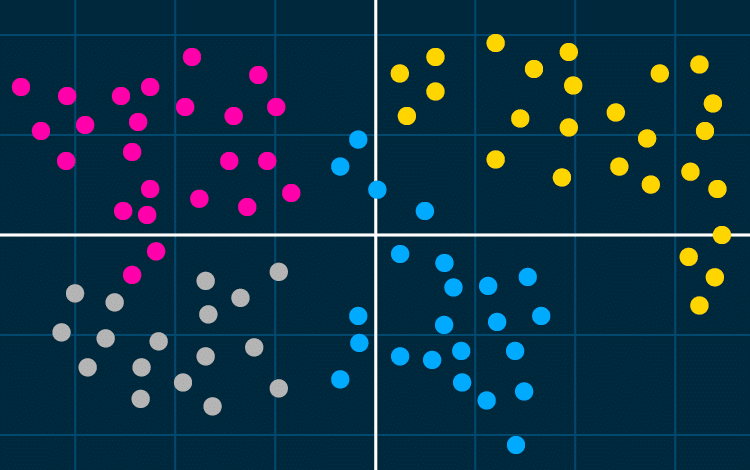
Customer Segmentation via Cluster Analysis
Cluster analysis uses mathematical models to discover groups of similar customers based on the smallest variations among customers within each group.
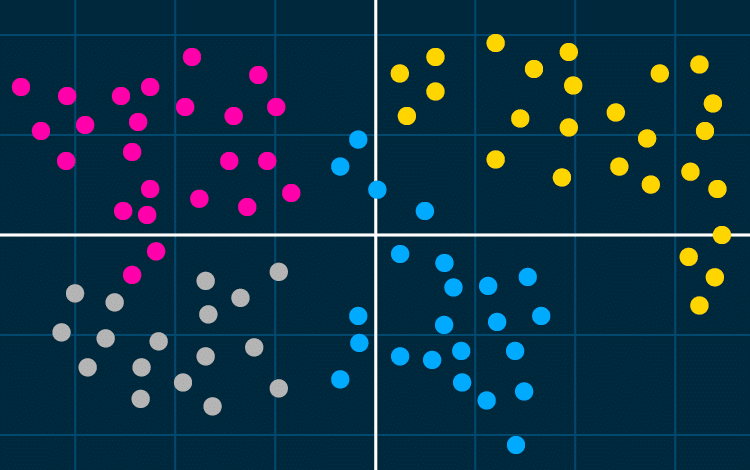
What is Cluster Analysis?
In the context of customer segmentation, cluster analysis is the use of a mathematical model to discover groups of similar customers based on finding the smallest variations among customers within each group. These homogeneous groups are known as “customer archetypes” or “personas”.
The goal of cluster analysis in marketing is to accurately segment customers in order to achieve more effective customer marketing via personalization. A common cluster analysis method is a mathematical algorithm known as k-means cluster analysis, sometimes referred to as scientific segmentation. The clusters that result assist in better customer modeling and predictive analytics, and are also are used to target customers with offers and incentives personalized to their wants, needs and preferences.
The process is not based on any predetermined thresholds or rules. Rather, the data itself reveals the customer prototypes that inherently exist within the population of customers.
What about Threshold/Rule-based Segmentation?
In threshold (or rule-based) segmentation approaches, the marketer selects a priori thresholds, typically in two dimensions, and divides the customers accordingly.
The disadvantages of this approach include:
- Thresholds are predetermined, leading to results that usually meet initial assumptions, as opposed to allowing the data itself to reveal the most meaningful divisions among the particular customer base being analyzed.
- There will be very large variances among the customers found in each segment.
- It is very difficult to perform the segmentation in more than two dimensions.
The following example illustrates why this segmentation approach is weak. Note the two highlighted customers – even though their purchase patterns are significantly different, they have both been included in the “yellow” segment.
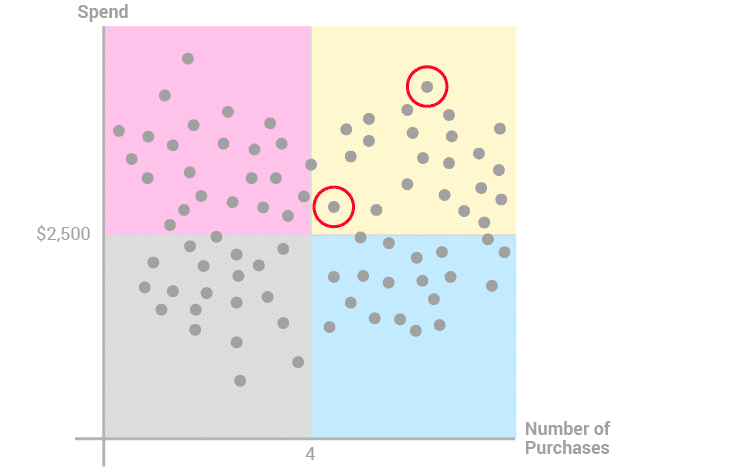
The Advantages of Cluster Analysis for Customer Segmentation
As compared with threshold/rule-based segmentation, the three main advantages of the analytical segmentation approach represented by cluster analysis are:
- Practicality – It would be practically impossible to use predetermined rules to accurately segment customers over many dimensions
- Homogeneity – Variances within each resulting group are very small in cluster analysis, whereas rule-based segmentation typically groups customers who are actually very different from one another.
- Dynamic clustering – The clusters definitions change every time the clustering algorithm runs, ensuring that the groups always accurately reflect the current state of the data.
In the following diagram, we see that cluster analysis identified five distinct customer personas in the same data set as above (the dots representing customers in each persona are colored differently). The customers within in each persona are very similar to one another and significantly different than those in other personas. In other words, each persona tells a different customer story.
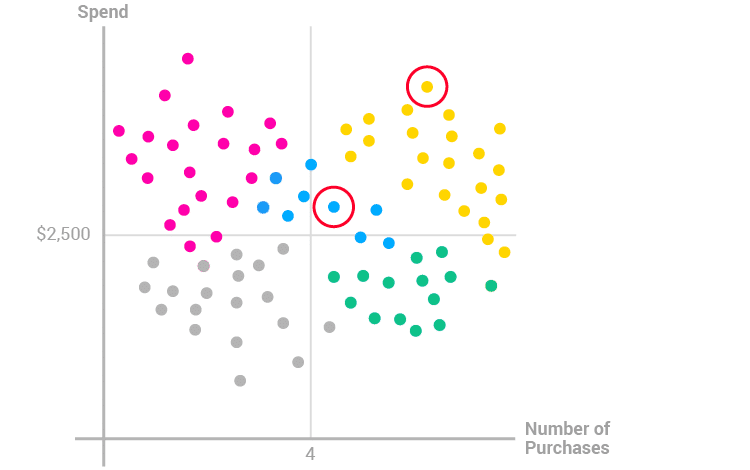
Unlike when the same customer sample was analyzed by threshold/rule-based segmentation, the same two highlighted customers are now properly segmented into different marketing clusters, or personas.
Sample Customer Cluster Analysis Result
The following chart shows the results of a three-dimension cluster analysis performed on the customer base of an e-commerce site. This analysis resulted in the discovery of four customer personas.
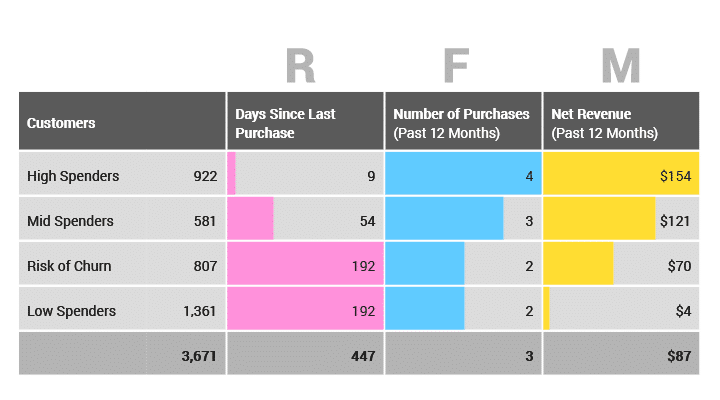
Once the store’s marketers have a clear view of the various customer personas, they are able to relate differently to each persona, with the marketing interactions most relevant to each persona’s product preferences.
In other words, the distinct customer personas discovered by cluster analysis allow marketers to model their customers and personalize marketing efforts for much greater effectiveness.
Closing the Cluster Analysis Marketing Loop
Because customer behavior changes frequently, performing cluster-based segmentation only once in a while is not sufficient. Ideally, it should be performed daily, taking advantage of all the latest customer behavioral and transactional data. For most online businesses, this means identifying dozens or hundreds of different personas that can be independently targeted by marketers. This, of course, is not something that can be easily done manually; rather, an automated system should be employed to ensure that the entire customer base is accurately segmented into relevant personas every day.
The next ingredient is connecting the discovered customer personas with the most relevant marketing interactions for each one. These interactions should cater to the specific wants, needs and preferences of each small, homogeneous group of customers represented by each persona. Marketing creativity must be mated with an automated multi-channel marketing execution system that will allow marketers to address any number of different personas with any number of different marketing campaigns, every single day.
Finally, there needs to be a measurement and optimization cycle in place. By scientifically measuring the results of each campaign in terms of monetary uplift, marketers can know which campaigns are working well and which ones need improvement. The end result will be highly relevant marketing communications – leaving no customer behind – that generate long-term customer loyalty, improved brand perception and maximum customer value.
No comments:
Post a Comment
Note: Only a member of this blog may post a comment.